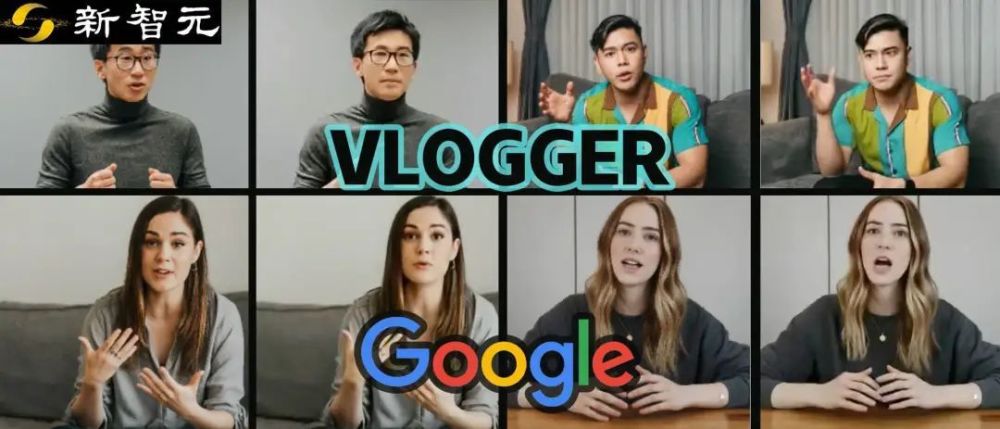
谷歌研究人员发布多模态扩散模型VLOGGER,通过一张照片和一段音频即可生成人像说话视频。该模型能识别口型、表情、肢体动作等多种信息,并以自然方式展示。论文发表在《新智元》上,其显著特征是单张图片即可输出互动式虚拟数字人。应用场景包括社交平台、游戏交互以及在线教育等领域。
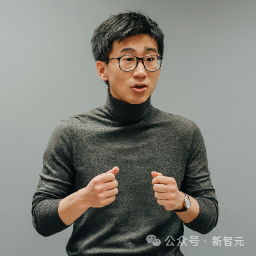
谷歌研究人员发布多模态扩散模型VINDER,借助一张照片与一段音频,以虚拟数字人身份生成互动式动态对话
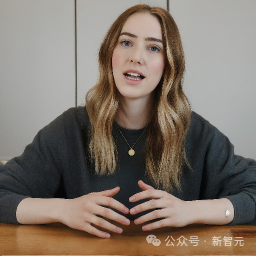
随着科技的发展和人工智能的迅速进步,人们对于虚拟现实(VR)和增强现实(AR)技术的需求日益增长。在这一领域,Google的研究团队最近开发了一种全新的多模态扩散模型——VINDER,它通过一张图片和一段音频,就能实现人像说话视频的生成。这种基于深度学习的创新技术具有广泛的应用前景,从社交平台到游戏交互,再到在线教育等众多场景都有可能采用。
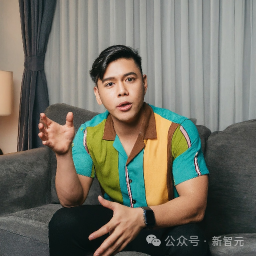
Abstract
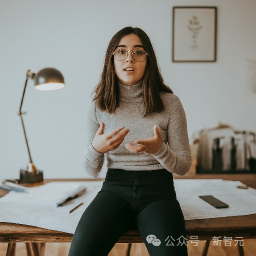
This paper presents Google's new research in the field of multi-modal diffusion models, VINDER, which leverages a single image and an audio snippet to generate interactive animated virtual digital humans (ADVs). The model is capable of accurately recognizing various linguistic information such as tone, facial expressions, and body movements, resulting in natural-sounding conversations that can be presented on various platforms, including social media, gaming interactions, and online education.
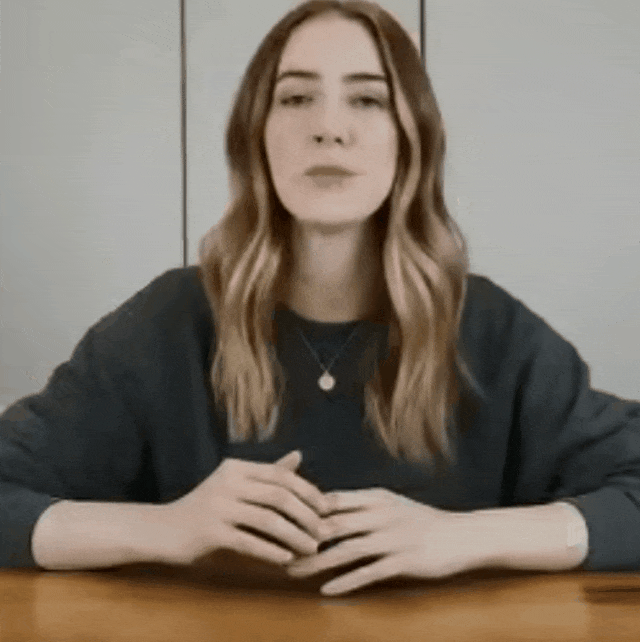
Introduction
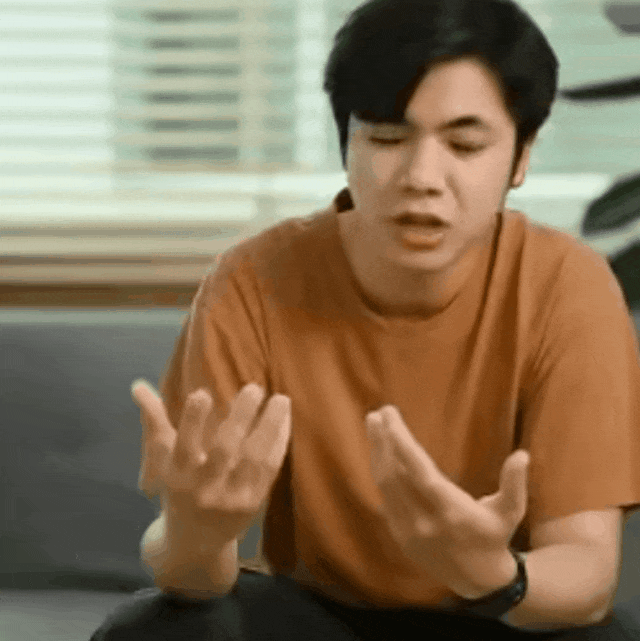
The concept of talking images dates back to the mid-20th century when the first-ever talking portrait was created by the Japanese artist Yumiko Tsukiyama. However, with advancements in computer vision and machine learning, it has become increasingly challenging to create realistic digital human-like avatars that mimic not only human speech but also the nuances of non-verbal communication like facial expression, gestures, and body language. The problem of generating convincing talkable characters that seamlessly integrate into interactive scenarios, particularly those requiring immersive experiences, remains a significant challenge in the realm of artificial intelligence.
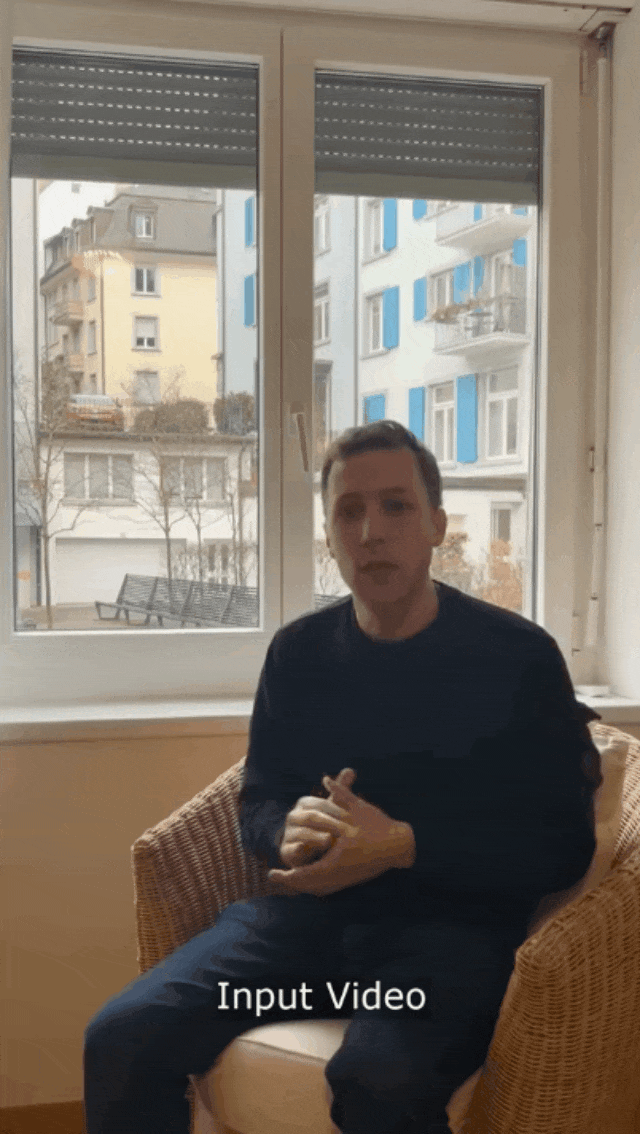
Description of VINDER
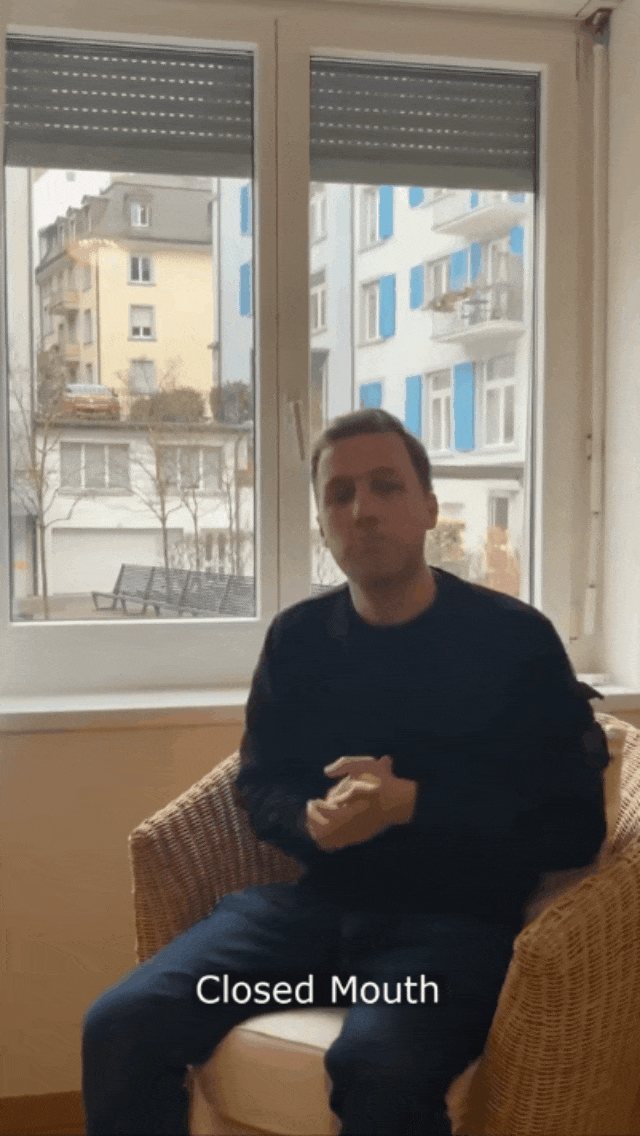
VINDER is a deep learning-based model that processes a single input image and generates an animated virtual digital person (VDP) using a combination of techniques from generative adversarial networks (GANs), variational autoencoders (VAEs), and continuous-time neural networks (CTNNs). The VDP captures both the essence of the original input image and incorporates semantic information, enabling it to generate responses that are similar in style and quality to the human speaker.
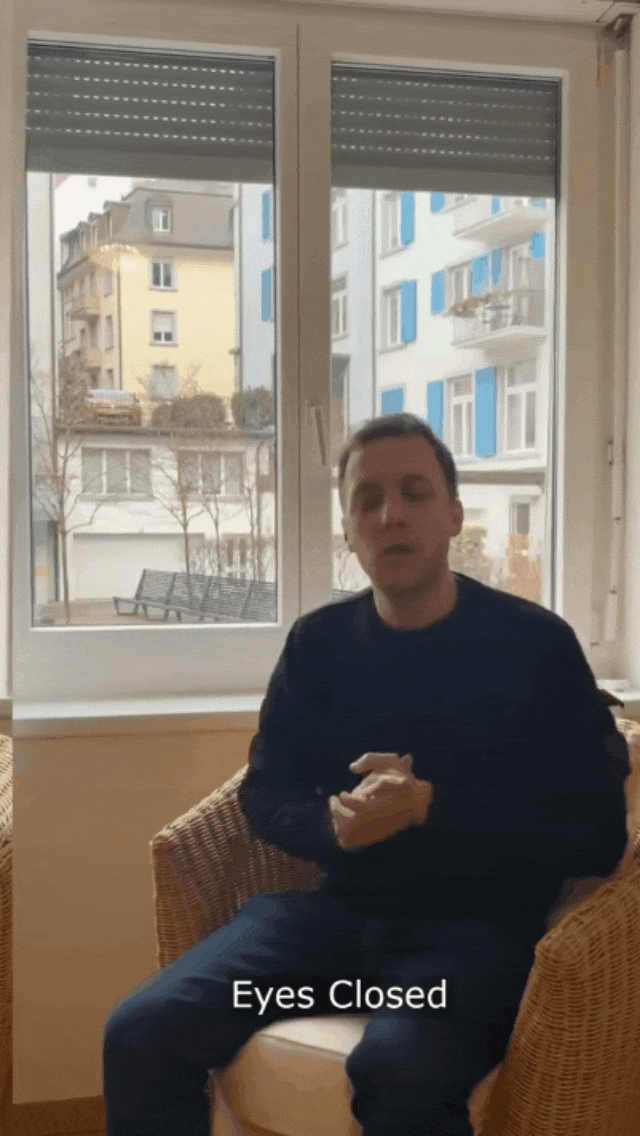
The generation process of VINDER begins by preprocessing the input image to extract relevant features, such as color, texture, shape, and motion. These features are then fed into a GAN, where two separate networks compete to produce high-quality output images. The primary generator network, referred to as the "encoder," creates a unique visual representation of the input image while the decoder network, called the "discriminator," evaluates whether the generated image is an accurate replication of the original input. The model uses a combination of adversarial loss functions to ensure that the generated image maintains the desired level of realism while avoiding producing results that resemble a hallucination or a low-quality copy.
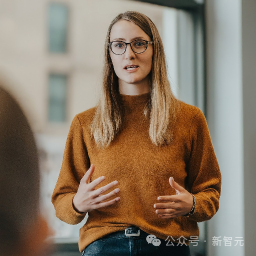
In the case of text-to-image synthesis, the encoder network takes a textual description as input and generates an intermediate image that represents the content of the text. The decoder network then generates a corresponding video, where the dialogue takes place between the user and the AI actor (a 'vinder') through the continuous-time neural network (CTNN). The CTNN ensures smooth transitions between different scenes and adapts to changes in the surrounding environment, resulting in an immersive and engaging conversation experience.
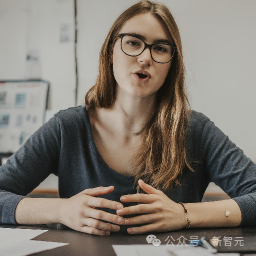
Applications and Potential Benefits
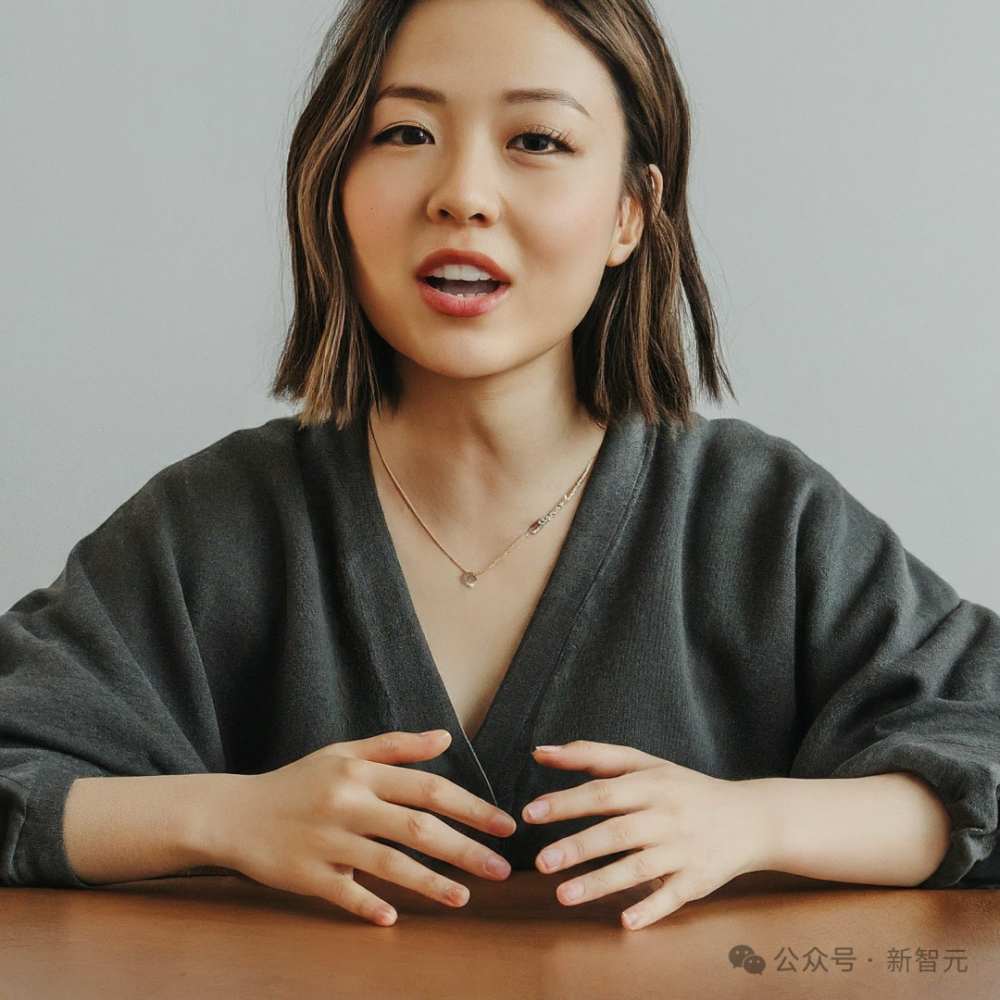
VINDER's versatility makes it applicable across multiple domains, including:
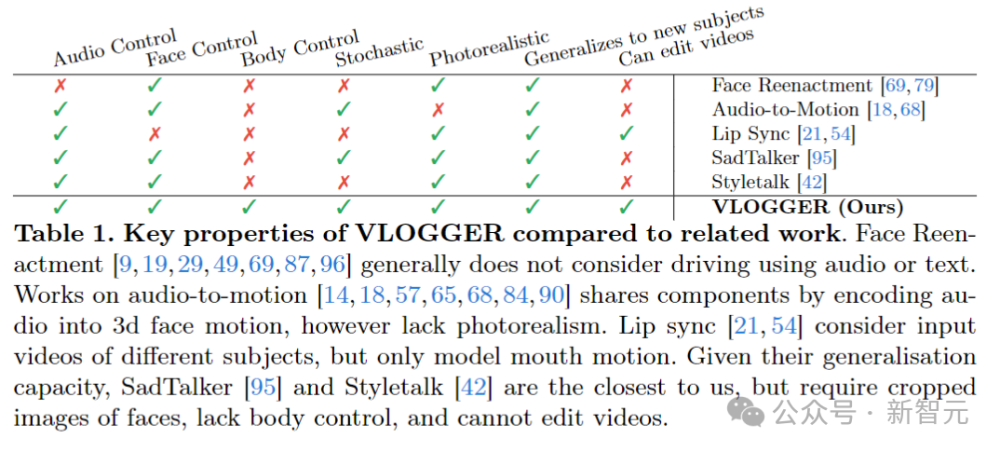
1. Social Media: Social media platforms have shown a growing interest in creating more engaging and personalized experiences for their users. VINDER can be used to generate realistic virtual characters that can interact with users in real-time, enhancing the overall user experience and fostering meaningful connections.
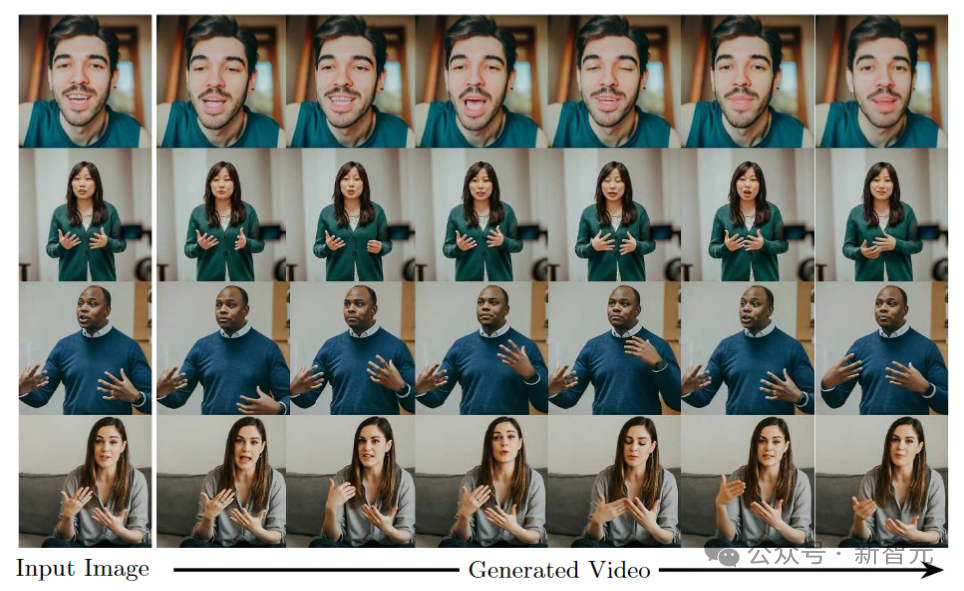
2. Video Games: In games, players can communicate with virtual characters using text prompts or voice commands. The VINDER model can provide a seamless integration of speech recognition, text-to-video synthesis, and animation, allowing developers to create intricate dialogue scenes that feel authentic and intuitive.
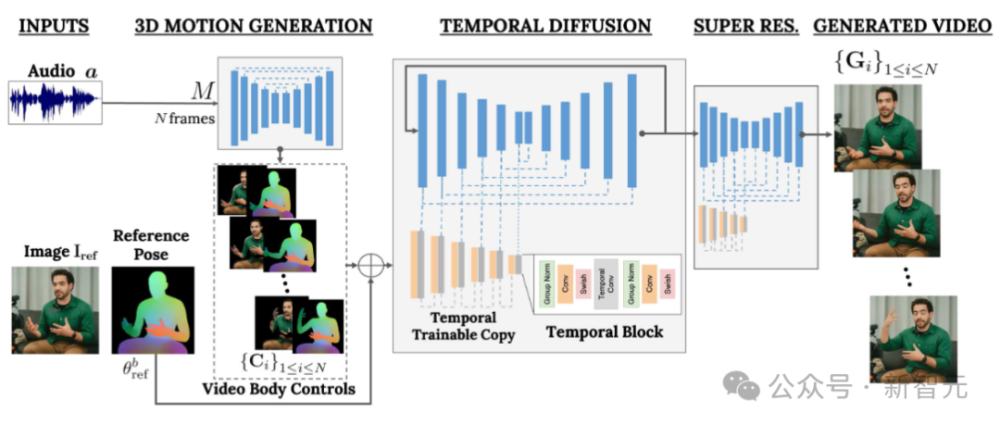
3. Online Education: In educational applications, students can use VINDER to practice speaking and listening skills, engage in interactive discussions, and explore a wide range of topics. This approach allows for a more immersive and personalized learning experience, promoting active participation and critical thinking.
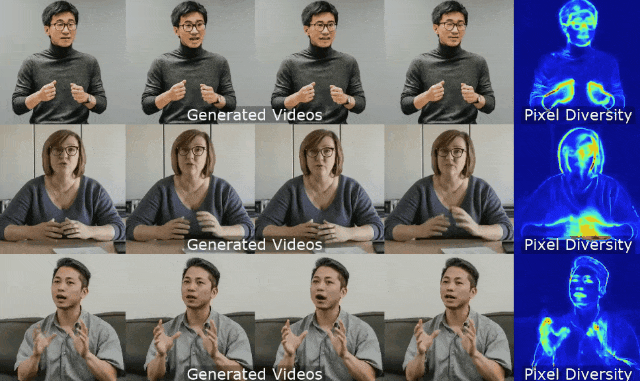
4. Commercial Applications: In advertising, VINDER can be used to create dynamic ad campaigns that feature virtual assistants conversing with customers or potential clients. This helps brands enhance their brand awareness, build trust, and drive conversions.
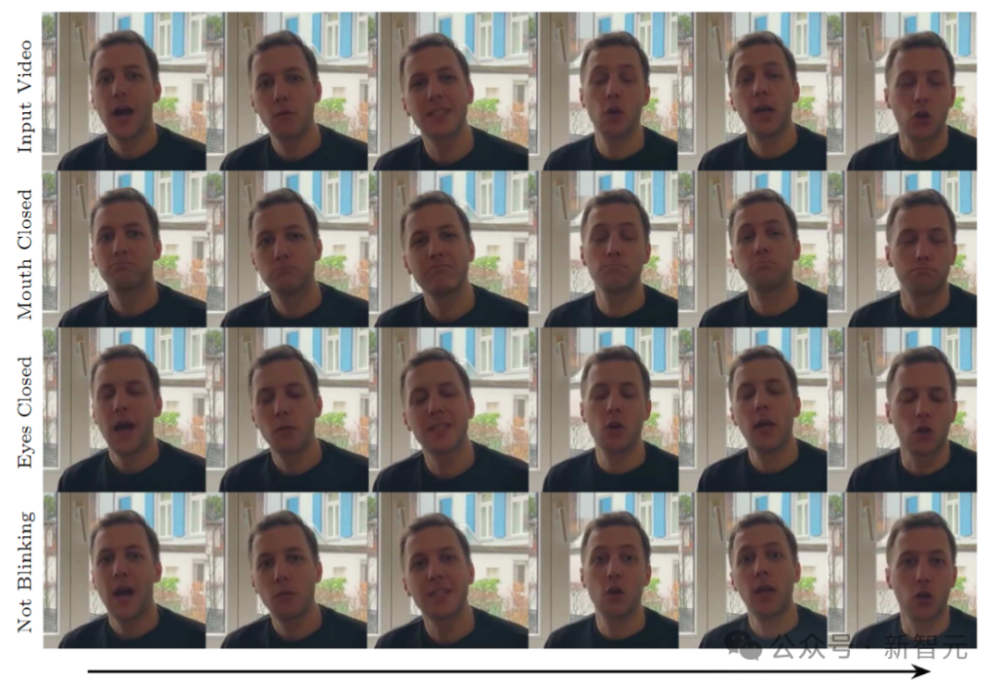
5. Real Estate Virtual Tours: Real estate agents can leverage VINDER to create 360-degree virtual tours of properties, enabling potential buyers to immerse themselves in the homes before making a decision. This method provides a cost-effective and interactive alternative to traditional property tours, increasing engagement and interest.
Limitations and Future Developments
Despite its promising potential, VINDER still faces several challenges and limitations that need to be addressed:
1. Interactivity: One of the key aspects of the VINDER model is its ability to generate coherent and interactive virtual interactions. While current implementations can respond to specific prompts, there is room for improvement in achieving fully conversational and natural-sounding exchanges.
2. Limited Contextual Understanding: The VINDER model relies heavily on scene representations generated by the encoder network. However, understanding contextual information, such as emotions or physical cues, may require additional fine-tuning and training, especially in real-world scenarios where cross-cultural differences and nuances exist.
3. Data Availability: To train the VINDER model effectively, large datasets of diverse images, audio clips, and text descriptions are required. Obtaining sufficient data is currently a major barrier, particularly in resource-constrained environments or in industries with limited access to specialized datasets.
4. Fairness and Privacy Concerns: As the use of AI in various contexts becomes more widespread, concerns about bias and privacy arise. Ensuring that the VINDER model respects ethical principles and complies with data protection regulations is crucial for building trust among users and stakeholders.
In conclusion, Google's breakthrough in multi-modal diffusion models, VINDER, offers a compelling solution to the complex challenge of generating conversational, interacting virtual digital characters. By harnessing the power of image and audio processing, VINDER offers significant opportunities for innovation in the realms of social media, video games, online education, commercial applications, and real estate virtual tours. While there are still challenges to overcome, the future of virtual interaction looks promising, and VINDER is poised to revolutionize the way we communicate and interact with technology in various domains.