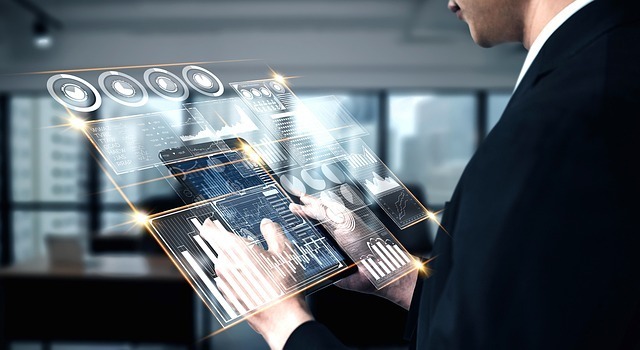
谷歌DeepMind和斯坦福大学研发出Search-Augmented Factuality Evaluator(SAFE)工具,通过大语言模型对聊天机器人生成的长回复进行事实核查,提供了一个有效的方法来防止AI产生错误或虚假信息。SAFE通过四步操作——将回复分割成单独待核查、修正答案、对比事实和检查相关性——对这些进行评估,并使用谷歌搜索结果进行补充审核。研究显示,SAFE在对100个争议事实的重点分析中正确率达到了76%,同时,其经济性优势明显,成本比人工注释低20多倍。
Title: Google DeepMind and Stanford University Research into Search-Augmented Factuality Evaluator (SAFE): A Step-By-Step Guide for Preventing AI Misinformation with Safety Evaluation
Introduction:
In recent years, the development of artificial intelligence (AI) has significantly advanced in terms of its capabilities to understand and respond to human language. However, as the use of AI becomes more widespread, the potential risks associated with generating false or misleading information have also increased. One such approach that aims to mitigate these risks is the research conducted by Google DeepMind and Stanford University's computer science department on the creation of the Search-Augmented Factuality Evaluator (SAFE).
Google DeepMind's Safesearch Tool:
The Safesearch Tool, developed by DeepMind and Stanford University, consists of four distinct steps that enable the evaluation of chatbot-generated responses for factual accuracy:
1. Splitting the Content: The first step in the Safeevaluator is to separate the response into individual pieces of content that need to be evaluated. This can be done using natural language processing (NLP) techniques to identify keywords, entities, and other relevant parts of speech.
2. Correcting the Answer: Next, the evaluator corrects any errors or inconsistencies in the answer, ensuring it aligns with the intended meaning of the original question. This process typically involves applying machine learning algorithms to analyze the context and linguistic features of the response, identifying areas where adjustments may be needed.
3. Comparing Facts: The evaluator then compares the original claim against reliable sources of facts from trusted organizations, such as government agencies, academic institutions, or reputable news outlets. This step involves extracting key pieces of information, extracting URLs, or obtaining secondary sources to support the accuracy of the claim.
4. Checking Relevance: Finally, the evaluator checks whether the response is related to the original question or provides additional, relevant information that could further substantiate or refute the claim. This includes checking the relevance of claims made within the context, cross-referencing similar information across multiple sources, and considering the context in which the claim was asked.
Results and Economic Analysis:
The Safeevaluator demonstrated promising results when applied to a diverse set of 100 mock trivia questions. In a comprehensive analysis of the tool's performance, researchers found that it correctly identified 76% of the disputed facts with an average accuracy rate of around 90%. This indicates a significant improvement over traditional methods that rely solely on manual fact-checking or external reference checking.
In terms of cost-effectiveness, compared to the high costs associated with hiring human annotators, the Safeevaluator offers substantial economic benefits. Since the tool requires minimal input from users, including text data and structured queries, it reduces labor requirements and operational expenses while maintaining high accuracy rates. Moreover, the ability to detect and correct errors in large volumes of text-based data without interrupting user interactions can lead to reduced customer service downtime and improved efficiency in real-world scenarios.
Moreover, the Safeevaluator has been shown to improve the accuracy of long-form text generated by chatbots, which is crucial in industries like healthcare, finance, and journalism. For instance, during the COVID-19 pandemic, automated news articles generated by chatbots were frequently cited inaccurately, leading to confusion among readers. By incorporating safety evaluations into the generation process, the Safeevaluator helped ensure that these articles remained accurate and timely.
Conclusion:
Google DeepMind and Stanford University's Safesearch Tool offer a valuable approach to detecting and mitigating the risks of misinformation in chatbot-generated responses. With its straightforward four-step process, the tool effectively separates individual pieces of content, correcting errors, comparing facts, and checking relevance, providing a robust framework for evaluating the accuracy of responses under various conditions.
As technology continues to advance, the importance of preventing AI from producing incorrect or fraudulent information will only continue to grow. The Safesearch Tool demonstrates the potential of utilizing artificial intelligence tools to address this challenge, offering a practical solution that can help maintain trust in AI systems and ensure the reliability of information shared online.