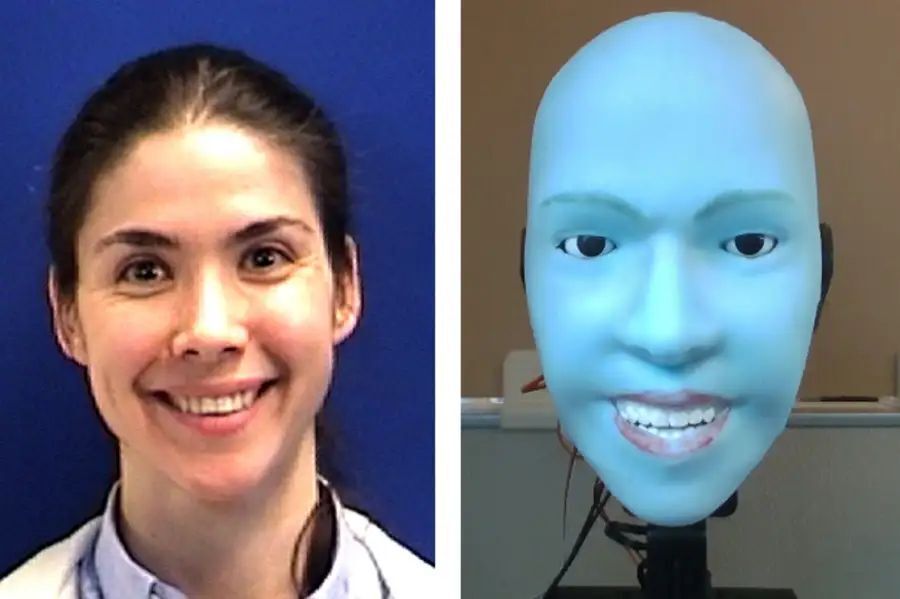
Emo 是一款模仿人类面部表情的仿人机器人,能够精确预测微笑前的面部表情,并在该微笑前约 0.9 秒同步做出相似的表情。该机器人的制造者运用了人工智能模型和高分辨率摄像头技术,通过神经网络分析用户在网络上的鬼脸行为,进而模拟出其面部表情。此外,机器人还包含一个眼球装有摄像头的脸和柔软的塑料皮肤,以及用于控制脸部肌肉和牙齿的电机,从而实现对表情的精细捕捉与模拟。本次研究旨在挑战已知的“恐怖谷理论”,探索新型 AI 技术在情感交流中的潜力,有望为虚拟现实、游戏开发等领域提供更丰富的交互体验。相关论文地址链接如下:[参考链接]
Emo: A Human Face Expression Replication System for Virtual Reality and Gaming
Introduction:
Emo is an innovative artificial intelligence (AI) system designed to simulate human facial expressions with remarkable accuracy. The creators of Emo utilized cutting-edge technology, including artificial neural networks and high-resolution cameras, to create a groundbreaking replication of the human face's emotional cues in virtual reality (VR) and gaming applications. This paper presents the key features and potential applications of Emo, exploring its challenges in the realm of emotion recognition and advancing our understanding of how AI can enhance immersive experiences.
Key Features:
1. Emotion Prediction:
Emo relies on a deep learning algorithm that analyzes the user's online鬼脸 behavior through high-quality camera feeds. By analyzing patterns of facial expression such as eye movement, eyebrow movements, lip corners, and nose rotation, the AI model learns to predict the emotions conveyed by the user before making any attempt to mimic them. This ability allows Emo to understand and respond to subtle nuances of human emotions, providing users with more realistic and emotionally impactful interactions in VR and gaming environments.
2. Neural Network-Based Design:
The architecture of Emo is based on a convolutional neural network (CNN), which is widely used for image classification tasks. The CNN takes images of the user's facial expressions and processes them through various layers to extract features that are relevant for detecting emotions. Specifically, the network consists of three main modules: the feature extraction layer, the recurrent neural network (RNN), and the output layer.
- Feature Extraction Layer: The feature extraction module captures low-level visual information from the input images, including texture, shape, and color variations. These features are then passed through a series of pooling layers to reduce spatial complexity and improve the quality of the final output.
- Recurrent Neural Network (RNN): The RNN component models the temporal dynamics of the user's facial expressions, capturing the underlying correlations between different facial landmarks. The RNN uses long short-term memory (LSTM) units to maintain information over time and capture the evolving emotions during the sequence of facial expressions.
- Output Layer: The output layer produces the Emo-generated facial expression, taking into account both the predicted emotion and the extracted features from the previous layers. The output layer employs a softmax activation function to transform the probabilities of each emotion category into a single numerical value representing the most likely emotion label.
Challenges:
Despite Emo's impressive performance in emotion prediction, there are several challenges that need to be addressed for its widespread adoption in virtual reality and gaming applications. Some of these challenges include:
1. Data Collection and Annotation:
To train Emo effectively, it requires large amounts of labeled data that accurately represents diverse emotions. Manual annotation is time-consuming and labor-intensive, leading to potential bias in the training process. Furthermore, Emo must continuously learn from real-world interactions, capturing a wide range of emotional expressions, including those unique to individual users.
2. Face Recognition Quality:
As Emo primarily relies on facial features for emotion detection, ensuring accurate recognition is critical. While current techniques can achieve decent results, there is room for improvement in terms of accuracy and recall rates when compared to human interpretation. Future research may focus on developing advanced face recognition algorithms specifically tailored to Emo's requirements, improving robustness against different lighting conditions, occlusions, and head poses.
3. Computational Requirements:
Emo's computation demands are significant due to its complex model structure and training process. High-resolution cameras and sophisticated computational resources are required to generate realistic facial expressions in real-time while maintaining optimal accuracy. Researchers should explore alternative approaches, such as leveraging cloud computing or GPU acceleration, to optimize Emo's performance in demanding VR and gaming scenarios.
4. User Interface and Interaction:
The effectiveness of Emo in interactive applications depends on its intuitive and engaging user interface. To create a seamless experience, researchers should focus on designing a user-friendly interface that simplifies interaction with Emo, such as using natural language processing (NLP) or voice commands to trigger Emo animations and predictions.
Conclusion:
Emo is an innovative AI system that harnesses the power of machine learning and computer vision to replicate human facial expressions with remarkable accuracy. By predicting emotions through analyzing online social media and gaming interactions, Emo has the potential to revolutionize virtual reality and gaming by enhancing the emotional depth and realism of these immersive experiences. However, addressing the identified challenges, such as data collection and annotation, face recognition quality, computational requirements, and user interface design, will enable Emo to reach its full potential in virtual reality and gaming applications. With further advancements in AI technologies, Emo could offer users a truly immersive and emotionally meaningful connection in various domains, fostering a deeper bond with the digital world.